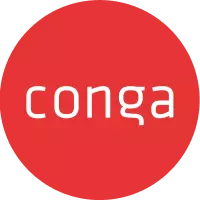
What is sales forecasting: best practices and tips
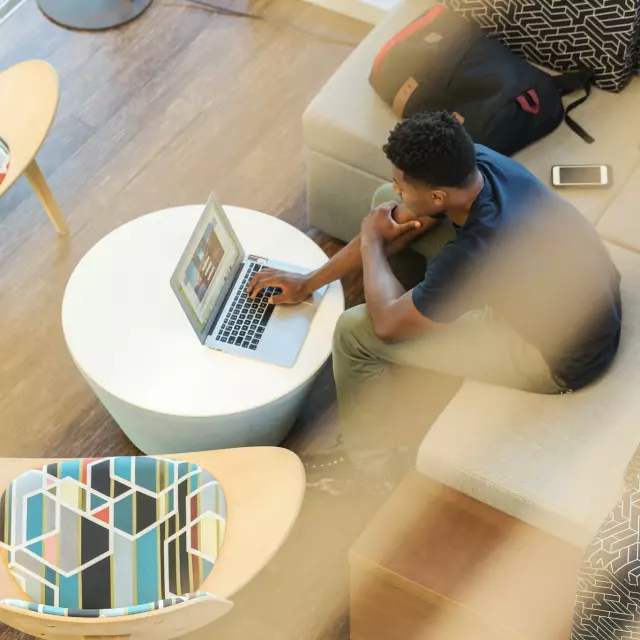
Sales forecasting is perhaps the most important tool for any sales rep or manager, as it shows them how well the company will do in the future. By using existing sets of data, the various sales forecast methods attempt to predict a company’s sales performance (expected demand) at the end of a measurable period. All types and sizes of companies rely on these forecasts to enable sales teams to make better informed decisions on resource allocation for the future.
Companies need accurate estimates of their future sales to help make choices concerning all aspects of a business. From purchasing to marketing all the way down to human resources, everyone depends on accurate sales forecasting to make decisions. Along with this, companies are measured against how well they perform compared to their forecasts. For this reason, it is extremely important that the methods and data used to create these reports are accurate. At the end of the day, these forecasts increase general knowledge, increase profitability, and reduce uncertainty.
Qualitative vs. quantitative sales forecasting
Forecast methods can be either qualitative or quantitative by design. Both of them have their own strengths and weaknesses, as well as usefulness. Quantitative forecasting methods use historical and current quantifiable data to predict future sales. They are able to take vast amounts of available data and translate them into future trends. Although there is a level of subjectivity involved (people subjectively decide which methods to use and which forecasts are best), the main objective of using quantitative forecasts is to be as objective about the future as possible. They attempt to remove the human element from the equation.
Qualitative (also called subjective) methods are more focused on short term projections and are generally broad in scope. The methods turn the opinions and knowledge of industry experts into formal reports, without implementing complex mathematical models. Qualitative methods are best used when there is no accurate or trustworthy historical data to input into models. In addition, as many quantitative methods use as an assumption that there will be no radical departure from the patterns of the past, it is best to tap into expert knowledge when those assumptions seem to be inaccurate.
As stated above, each of the two methods have their advantages and disadvantages. It is up to each individual company to figure out which suits them best. Qualitative methods are best when there is not any past, reliable data laying around. However, they rely on experts’ objective opinions (subjectivity cannot be completely removed) and are unable to process large amounts of complex information. Quantitative techniques are best used when there are large data sets available to analyze, however fail when the market experiences a change that has never happened before. Overall, it is best to use a combination of both methods to cover all the bases. Supplement initial quantitative analysis with qualitative opinions from experts to make changes based off of information that was not initially considered.
Two types of quantitative forecasting
Time series model
These models exclusively use past experience and historical data to predict future sales. Although the amount of time available to base the model off of may vary from company to company, it is incumbent on the forecasters to gather as much data as possible to eliminate overlooking important trends. These methods are fairly simple to employ, and can be used by any company that has access to enough data. However, one of the main problems of these methods is that they rely exclusively upon the past to predict the future. They do not take into account possible shifts that may be occurring or look like they will occur. The models do not take into account external factors such as price changes, competitors’ actions, or economic conditions.
Causal model
Causal methods of forecasting attempt to identify the underlying factors that could possibly be influencing the future sales numbers. These indicators can predict future changes in demand, and rely on a cause and effect relationship between the independent and dependent variables. In sales forecasting the independent variables might be things like price or economic conditions, while the dependent variable is future sales. The independent variables cause the dependent variable to fluctuate. By using this method companies may find that their monthly sales are directly linked to a few independent variables, such as GDP growth or inflation. This method is best when a company’s sales vary widely depending on unknown stimuli.
Common sales forecasting methods
1) Jury of executive opinion (qualitative)
This method is when a group of executives from the major stake-holding functions (marketing, sales, finance, etc.) around a company come together to create a general forecast. This is one of the most common forecasting techniques used, as it is quite simple to perform.
Pros: Quite efficient in forecasting potential changes to demand patters. Also valuable when there is limited historical data.
Cons: Subject to the opinions and prejudices of the executives creating the forecast.
2) Delphi method (qualitative)
Step one of the forecast entails each member of a panel creates a forecast and their reasoning behind it. The anonymous answers of each panel member are then summarized. Step three gives each panel member a chance to re-evaluate or maintain their original forecast.
Pros: Allows each member to develop their own reasoning without influence from other members.
Cons: Again, this relies on the opinions of experts and can therefore be unreliable. It relies on a certain level of expertise for each individual member.
3) Sales force composite (qualitative)
This technique uses the experience of the sales teams (individual sales reps, managers) to produce forecasts. It is a grass roots method that attempts to gain insight into regions, products, and even individual customers.
Pros: It incorporates the knowledge of the people that are closest to the customer.
Cons: Quality of many sales people inputs can be of poor quality, and many companies need to implement strategies to invest sales people into making accurate, quality reports.
4) Moving averages (time-series)
This method averages out data in a time series model. The longer the time series the smoother the average. It is used to smooth out short term fluctuations and extrapolate into the future.
Pros: Creates a new data set with much less variation. Good for forecasting far into the future.
Cons: This method should not be used in the short term by companies that experience a strong variance in month to month demand, or companies that experience seasonal patterns.
5) Exponential smoothing (time-series)
This method is another method of smoothing out existing variations. Essentially, it gives varying weightings to each of the different data sets on which the forecast is to be modeled.
Pros: It is relatively simple compared to other quantitative forecasting methods, and requires only a limited amount of data.
Cons: Again this method does not adjust for trends or seasonal changes in demand.
6) Leading indicators (causal)
This is the use of mathematical equations to establish a relationship between the dependent variable, and one or more independent variables. It depends on these relationships being consistent over time, and uses the data to estimate the performance of other variables.
Pros: These models are able to look at relationship between the factors that influence demand.
Cons: Assumes the relationships between indicators to stay consistent over time.
7) Econometric modeling (causal)
As opposed to the indicator approach, this modeling does not assume relationships remain the same. These models mathematically test the strengths of these relationships between variables over time.
Pros: Able to test for variance in the relationships between variables over time.
Cons: Extremely complex to implement and interpret.