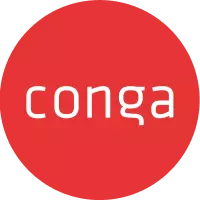
Achieving predictable revenue: fixing your quote-to-cash process
Predictable revenue is a hot topic for companies who want to better understand and grow their sales funnel. But a lot of data goes into creating a predictable revenue model—some of which might be easy to find, and other points may not exist yet.
When you have the right data for a predictive revenue framework, you can better understand historical deals, identify your margin sweet spot, and optimize your pricing strategy to create better pipeline predictability.
In this on-demand webinar, you’ll learn:
- What data points are valuable to track and how to collect them
- How to find insights into your data
- The best way to scale a predictable revenue framework as you grow
How to achieve predictable revenue through CPQ
Predictable revenue is a hot topic for enterprises who want to better understand and grow their sales funnel. But data is a key consideration for creating a predictable revenue model. When you have the right data for a predictive revenue framework, you can better understand historical deals, identify your margin sweet spot, and optimize your pricing strategy to create better pipeline predictability.
Conga’s VP of Product Marketing for CPQ, Amol Baviskar, and Senior Director of Sales Ops, Tom Mirrione, discuss what data points are valuable to track, how to collect them, how to find insights into the data, and the best way to scale a predictable revenue framework as you grow.
The value of predictable revenue for businesses
It’s challenging to run a business without predictability. As Tom puts it, it’s a key focus of revenue operations teams and, “we do everything in our power to drive both growth and predictability.”
At the heart of both growth and predictability is pipeline data. Some of the classic questions we see in revenue operations that we need this pipeline data to answer include:
- How are we tracking to plan?
- What’s the gap in meeting the quota?
- Do we have enough pipeline to cover the gap?
Most organizations look at historical pipeline conversion rates to answer these questions. However, there are many dimensions to the data that impact these answers – —a lot of which aren’t tracked:
- Intent
- Channel
- Average deal size
- Deal duration
- Geography
- Segment
- Industry
- Product
The ability to slice and dice pipeline data along these various dimensions is critical to building a predictable model. And of course, without a good model, it’s tough for a business to get in front of risks and course-correct before they become real issues.
The value of predictable revenue for the customer
Predictable revenue impacts the customer experience too. Amol states that the critical challenge is inconsistency. When there are inconsistencies in the product configuration, price estimation, and quoting process, trust and brand issues result. He gives two examples from two different industries:
“I recently heard from the CRO (Chief Revenue Officer) of a small but high-growth company with $20M in revenue. He mentioned their salesperson presented a $20K proposal to a customer in 2020. The deal went on hold during COVID-19, and in the meantime, the salesperson left the company. Recently, the customer re-engaged, and a new salesperson proposed the deal at $60K. The customer was shocked to see the price triple for the same product. The company didn’t have a systematic way to track proposals, resulting in a significant inconsistency. The prospect lost trust, and the company lost the deal. The company’s inconsistency resulted in a poor customer experience.”
“As another example, we often hear from large companies that different business units use different processes and CPQ tools. Their customers complain that it’s challenging to negotiate in separate ways with two business units of the same company. They might as well buy products from two different companies. Again, this results in delays or losing deals due to inconsistent quoting processes across business units.”
Inaccuracies are frustrating, causing delays and customer satisfaction issues. These negatively impact the customer experience, business growth, and predictable revenue.
Creating predictable revenue through CPQ
To achieve predictability, it’s imperative to focus on business procedure. “Without solid foundational processes, it is simply impossible to scale effectively, in my view,” Tom states. He suggests that businesses lead with process changes and then layer on the technologies and automation needed to support and streamline them.
For example, the tasks applied to manage SKUs, price books, configuration rules, and discount approval directly impact pipeline data. That’s where CPQ steps in to act as an accelerator and a solution to enforce said processes. CPQ allows you to implement the necessary steps that result in:
- Natural cross-selling of complementary products through bundles and promotions.
- Valid configurations that work for customers and the manufacturing floor.
- A pricing engine that delivers rule-based discounting and the corresponding review and approval process.
Plus, CPQ is foundational to predictive models. It creates a repository of configurations and discounts that can be tied to deal attributes, like competitors, segment, industry, deal size, and win/loss data. You can start probing the data to answer questions like:
- What configurations and combinations typically sell together, and at what price points?
- Can we deliver standard, reusable configuration and discounts as a starting point to reduce the time it takes to generate a quote?
- How long does it take to turn around a quote approval?
- How much variation do we see in terms of discounts? Can we auto-approve certain discount thresholds?
- Can we sell standard, simple configurations with an online, self-service e-commerce solution?
- Are we doing low margin deals to win the opportunity? Are those deals worth doing?
With the answers to these questions, you can start surfacing the characteristics of historically successful deals, position complementary products, and optimize discount levels with recommendations at quote time for all your in-flight opportunities. These outcomes can maximize revenue, margins, or a combination of the two. While these models are compelling, they require the data repository that CPQ creates.
CPQ processes as predictable revenue drivers
CPQ is comprised of three main processes: configure, price, and quote. Tom and Amol dive into what data each component of the tool provides and how it drives predictable revenue.
Proper configuration drives predictable revenue
In CPQ, product configuration sets the foundation. You must understand the customer’s wants and needs to produce suitable offerings and solutions. You must also ensure you build accurate configurations to remove any downstream problems.
The configuration process varies depending on the complexity level of your overall offerings and customer needs. Amol gives the example of a highly complex configuration like network equipment for a data center. Configurations for a data center could require a lengthy process of figuring out the right combinations.
Instead, CPQ provides a library of complex configurations that are tagged with the business need each solution solves. This creates a starting point for similar needs in the future. This library is crowd-sourced from all sales users.
Data-driven pricing strategy drives predictable revenue
CPQ is more than a pricing engine to fit a prospect’s budget or to apply modest discounts through rounds of negotiations; as you accumulate all the transactional data, building models that optimize pricing becomes possible.
Let’s say you are quoting a solution for an enterprise customer in the high-tech vertical and competing against two other companies for a deal around $200K. With CPQ, you can look at historical pricing data for prior deals with those same characteristics and target a sweet spot based on the pricing applied in winning contracts. Ideally, it’s a spot that ensures you’re not over-discounting and eroding margins but also not under-discounting and proposing a high price relative to the competition.
Another advantage is pricing more consistently, which is vital because your buyers will backchannel their research by reaching out to their network once they have a proposal. It also mitigates the kind of wild swings that erode customer trust.
Ideally, the business would recommend the target price range at quote time. Still, at a minimum, the model could be used to define discount approval thresholds or even auto-approve discounts within the target range.
When you start to leverage the data this way, it’s a win for the sales reps, an operational win for the extended team, and a win for the bottom line.
Accurate quotes drive predictable revenue
Quoting is a process where the actual back and forth happens with the customer from sending out a budget or approved quote. There are multiple aspects including finalizing payment terms and conditions.
Quotes can get hung up when each product configuration or pricing stipulation must be approved by legal. It’s crucial to ensure that one process does not block the other. The proposal-to-contract negotiation process should be practical to avoid delays and lead to a more consistent timeline for revenue. Focus on creating pricing and product configurations that are pre-approved by legal to reduce the number of contracts they need to approve.
Business outcomes of predictable revenue
Now that we’ve covered how data-driven strategies grow revenue and scale the business and how CPQ supports that, predictable revenue will create the following key business outcomes:
- A better understanding of your pipeline
- Higher forecast accuracy
- Data to set meaningful, achievable targets for product-specific pipeline and bookings
- Optimized deal structure based on revenue or margin
- Reduced SKU proliferation
- Improved transaction velocity
- The ability to anticipate problems with enough time to course-correct
- Improved brand value by providing a top-of-the-line experience throughout all interactions.
- Tailored engagement based on previous interactions and purchase history
- Predictable billing and payments, helping the business’s cashflow
- Streamlined renewals
While CPQ is a technology that drives operational efficiency through automation, there is much to be said about the value of rules-based configuration, discounting, and approvals. It’s essential to think about how these capabilities allow you to run more efficiently and deliver a plethora of data to drive predictable revenue.
When you combine configuration and pricing data with pipeline data, you can build models that offer the essential insights to achieve that goal of predictable revenue.
If you’re interested in learning more about CPQ, check out our Ultimate Guide to CPQ.